AI Polling Product
Our AI polling strategy results from rigorous testing and experimentation over several successful election cycles, shown in Figure 1. It is a cutting-edge system that utilizes a powerful ensemble of state-of-the-art machine-learning algorithms. Its meta-learning approach (few-shot learning model) allows it to rapidly learn from small amounts of data, making it highly adaptable to new polling environments and capable of accurate predictions even without extensive training data. The deep reinforcement learning component optimizes its behavior based on feedback, enabling autonomous performance improvement over time. This adaptability allows the system to stay up-to-date and generate reliable predictions for different elections.
Three key components
1. Data Gathering: We collect and integrate various data sources, such as social media, polls, and news, to provide a comprehensive understanding of the current political landscape. We currently have access to Facebook data, Twitter data, and Reddit. We are working to integrate other platforms like Quora, Instagram, and TikTok.
2. Data Transformation: We transform unstructured data such as text, audio, and images into structured data for analysis. This process involves natural language processing, computer vision, and network theory techniques. For example, video-to-text algorithms allow us to get the text of a video speech, while face recognition algorithms allow us to get the age and gender of the audience (when possible).
3. Machine Learning Algorithms: Our system employs state-of-the-art machine learning algorithms to generate accurate predictions and insights about public opinion toward political candidates. These algorithms include:
- BERT for Sentiment Analysis: By using BERT for sentiment analysis, our system is able to more accurately gauge the intensity of support or opposition towards a candidate for a given text, which is crucial for generating accurate predictions. BERT is also highly adaptable, which means it can quickly adjust to new data sources and languages, allowing us to provide comprehensive sentiment analysis across different regions and languages.
- Topic Modeling and Clustering: Advanced natural language processing (NLP) techniques, such as topic modeling and clustering, allow the algorithm to identify the key issues and themes present in large volumes of text data. By understanding which issues are most important to voters and how they may impact candidate support, we can generate a more nuanced understanding of public opinion. This enables our system to generate more accurate predictions and recommendations, which can be highly valuable for political campaigns seeking to refine their messaging and target specific voter groups.
- Multi-Parameter Adaptive Weighing System: A multi-parameter adaptive weighing system model is designed to work by considering the traditional poll predictions as an initial condition of the weights. It uses reinforcement learning to optimize such weights and better understand the variations in public opinion towards a candidate over time by considering the social information collected for each candidate, such as reactions, retweets, Google searches, news, key issues, themes, etc. This allows our system to adjust its estimates dynamically and improve its performance over time.
- Graph Neural Networks (GNN): All the information from the above sources converges into a GNN model, the final component of our AI polling strategy, which weights all this information to estimate the share for each candidate. This approach effectively identifies patterns and relationships in large datasets, enabling the system to generate highly accurate and reliable predictions.
- Homophily detection. Through homophily detection, the algorithm estimates the likelihood that an undecided user supports one of the candidates.
Overall, our AI polling strategy leverages the latest advancements in machine learning, including few-shot learning, DRL, BERT, topic modeling, clustering, multi-parameter adaptive weighing system, and GNN algorithms, to provide highly accurate and reliable estimates of public opinion towards political candidates.
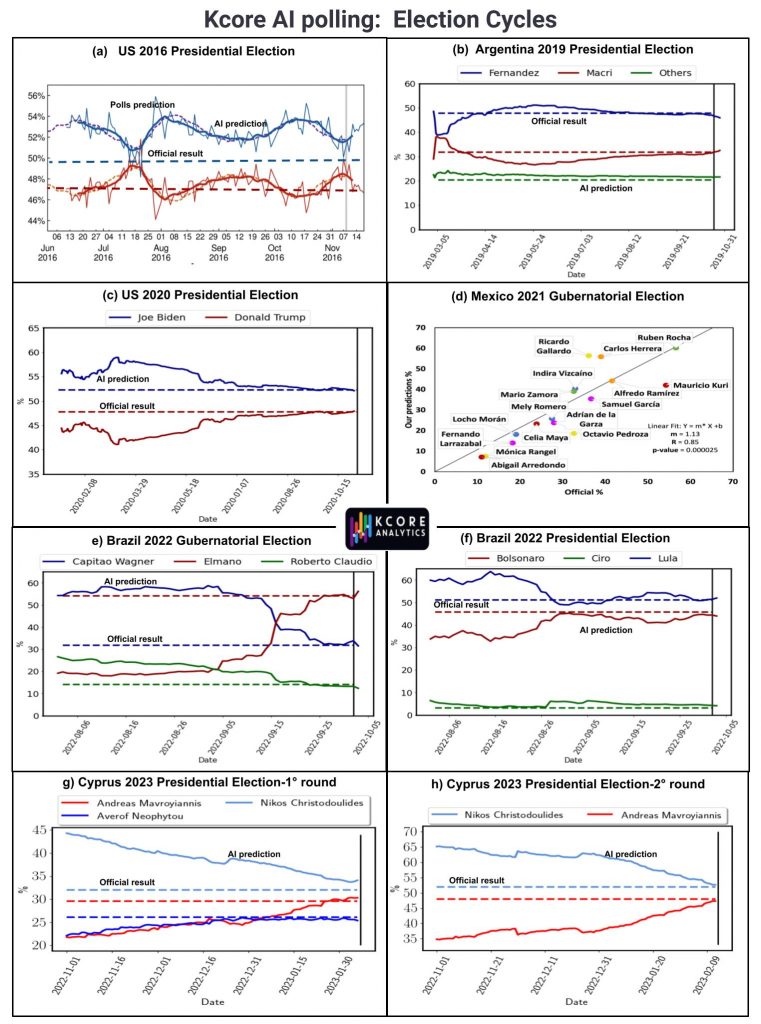